Statistical Analysis on USA Youtube Trending Videos
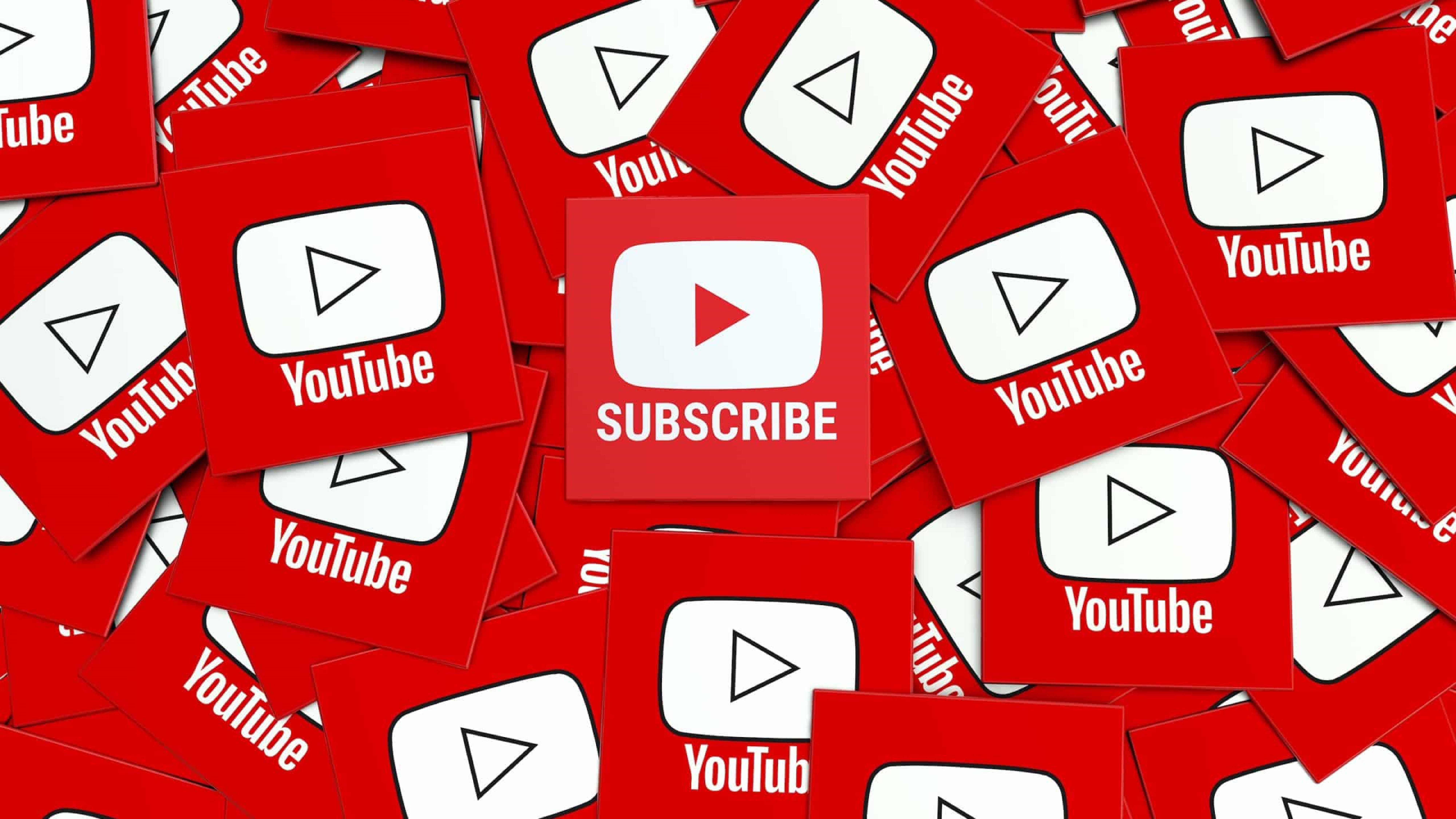
Problem Statements
The background of the problem is very important to know because it will make it easier to identify the source of the problem and determine the right solution. Background information on the problem can be used as a basis for evaluating data and making wise decisions in dealing with the problem at hand. In this context it includes:
The hypothesis being tested is the anti-thesis of the problem background. Everything about the data has no significant intercorrelation and even if there is it is just coincidence.
Data Understanding
This dataset is provided as material for working on the topic of Trending Video Statistics on YouTube specifically for the United States region. In the early stages, the information contained will be described in more depth to understand its characteristics. As material for analysis, the datasets used are sourced from following link, for each row the data contained consists of 16 columns, each of which contains information as follows:
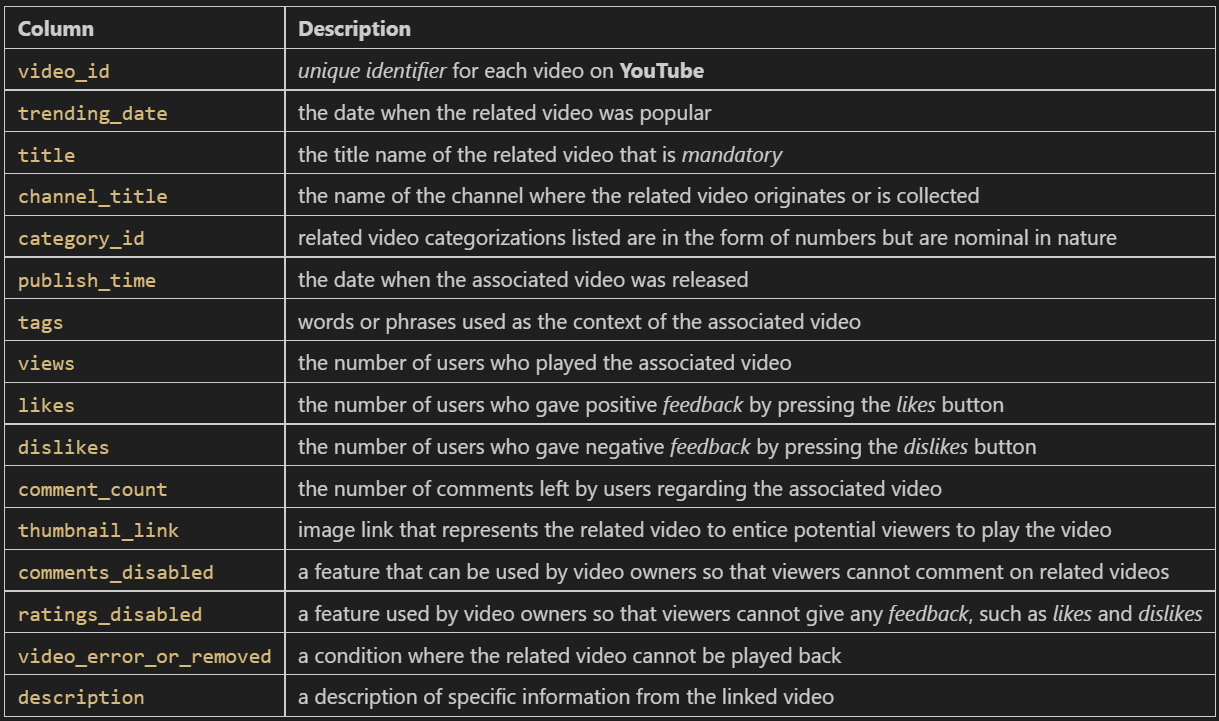
The dataset will go through some kind of data cleansing and feature engineering processes in order to make it well-prepared before taking into account of Exploratory Data Analysis.
Explanatory Data Analysis
Views Research
The analysis in this section is to identify impression metrics based on data in the views column. Here it will be examined whether there is a significant difference between the average number of views in each video category.
Engagement Importance
Engagement is a term used to describe the interaction between viewers and uploaded content. It includes various things such as the number of likes, dislikes, and comments. Here the data will be filtered based on the feature availability status in ratings and comments.
Growth Analysis
Growth Analysis is a process of measuring and evaluating the growth of an aspect. This analysis can provide valuable information to improve YouTube service performance and predict future growth. By knowing the growth trends in each category, YouTube can determine a priority scale to make recommendations regarding which industries are currently popular.
Engagement Rate Trends
After understanding the significant relationship between the number of views and engagement. We will try to analyze turnover from each increase in views to the number of engagements in terms of engagement rate.
Decline in Engagement Rate
That way, we will discuss this phenomenon in depth according That way, we will discuss this phenomenon in depth according
Days-to-Trending
Days-to-trending is a metric used to measure the time it takes for a video to enter the trending list on YouTube. The calculation is done by calculating the difference between the date the video was uploaded and the date the video was first trending.
Trending Duration
Trending on YouTube refers to videos or content that are currently popular at a particular moment. Trending is calculated based on a number of factors, including the number of views, level of engagement, and speed of growth of views. YouTube has a special section for content that is trending, which users can see when opening the main page or doing a search on the platform.
Video Quality
The quality of a video in this discussion is based on engagement rate which develops every time it is trending. The quality of a video can change depending on how big the effect of decline is received. Therefore, the categorization will be made with the following provisions:
Sentiment Ratio
Sentiment ratio refers to the ratio of positive and negative sentiments expressed in a video. This ratio is calculated by dividing the number of negative sentiments by the positive sentiments expressed. Sentiment ratio can assist in evaluating audience response and can be used to measure the popularity or success of a product, brand, or campaign embedded in a circulating video.
Similarity Degree
Similarity degree is a measure to measure the extent to which two or more objects have the same context in terms of certain characteristics, features or characteristics. This calculation is obtained from a comparison of the number of common tags of the total tags used by a video.
Recommendations
It is necessary to evaluate all insights that have been generated in the Exloratory Data Analysis section, which are as follows:
intentionally left blank
Please find codes detailed on Github channel.